.png)
Data Talks on the Rocks
Data Talks on the Rocks 1 - Modal Labs, Pinecone, Ask-Y
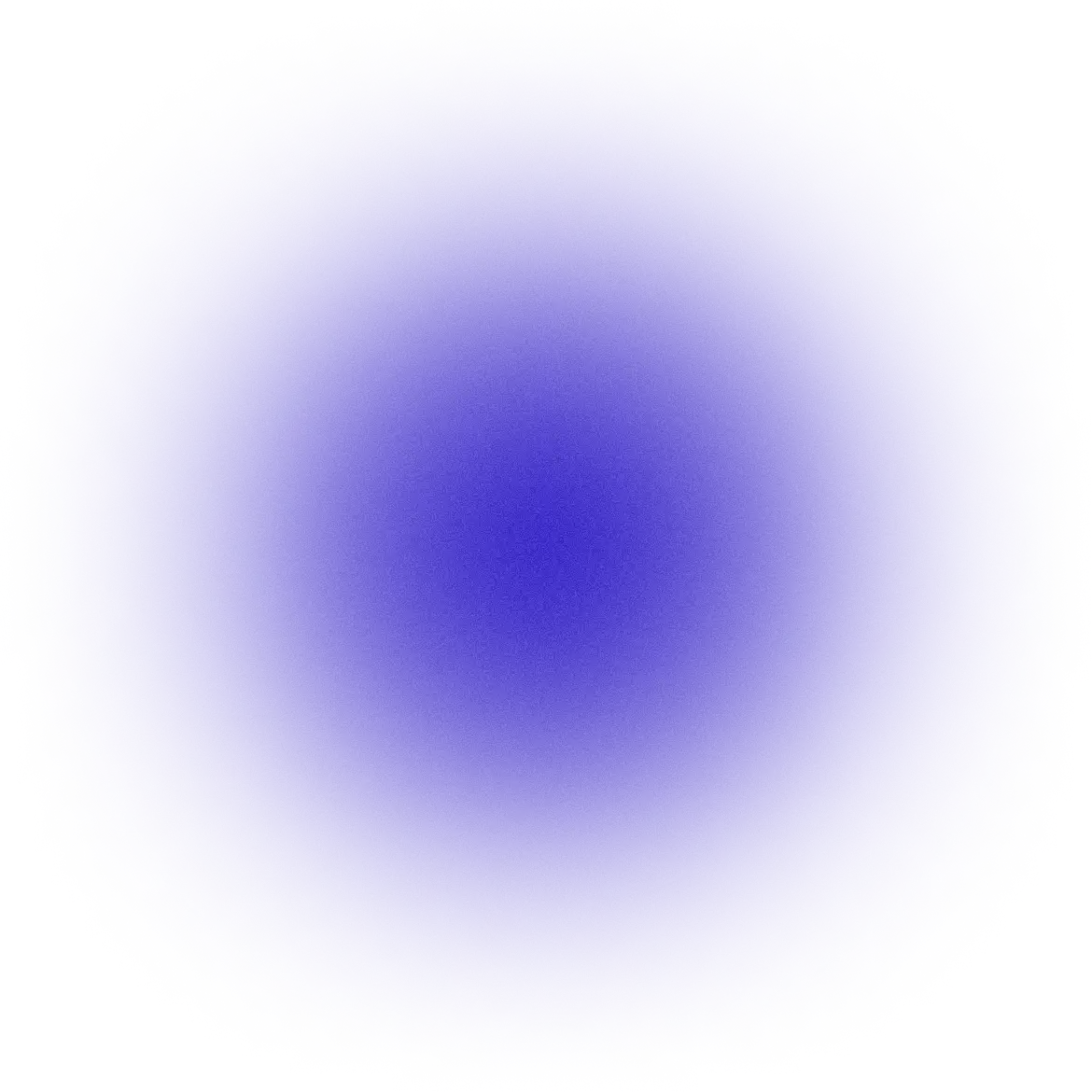
CONTENTS
Data Talks on the Rocks is a series of interviews from thought leaders and founders discussing the latest trends in data and analytics.
Data Talks on the Rocks 2 features:
- Guillermo Rauch, founder & CEO of Vercel
- Ryan Blue, founder & CEO of Tabular which was recently acquired by Databricks
Data Talks on the Rocks 3 features:
- Lloyd Tabb, creator of Malloy, and the former founder of Looker
Data Talks on the Rocks 4 features:
- Alexey Milovidov, co-founder & CTO of ClickHouse
Data Talks on the Rocks 5 features:
- Hannes Mühleisen, creator of DuckDB
Data Talks on the Rocks 6 features:
- Simon Späti, technical author & data engineer
Data Talks on the Rocks 7 features:
- Kishore Gopalakrishna, co-founder & CEO of StarTree
Data Talks on the Rocks 8 features:
- Toby Mao, founder of Tobiko (creators of SQLMesh and SQLGlot)
- Jordan Tigani, co-founder of MotherDuck
- Yury Izrailevsky, co-founder of ClickHouse
- Kishore Gopalakrishna, founder of StarTree

Two weeks ago I was lucky enough to bring together a few of NYC’s data, analytics, and AI luminaries for a discussion in a unique backdrop of Manhattan’s oldest distillery. Our esteemed panelists included:
- Edo Liberty is the founder & CEO of Pinecone, the leading vector-database-as-a-service offering (which has raised a total of $100mm), and a former professor of computer science. When not working, coding, or teaching, Edo is an avid surfer.
- Erik Bernhardsson is the founder & CEO of Modal Labs (which announced its $16mm Series A this week), a service which simplifies how developers scale workloads in the cloud. Fun fact, Erik has never owned a TV or a car.
- Katrin Ribant is the founder & CEO of Ask-Y, and the former founder of Dataroma, which sold to Salesforce for nearly $1B. When not building start-ups, Katrin can be found kite surfing in the Caribbean or Greece.
The full transcript of the talk can be found here, but I’ve noted some of my favorite highlights below.
- Erik discusses why he was crazy enough to start up yet another data infrastructure company in the context of hundreds of existing players, and what Modal Labs original goals were - check out the queued up video here.
- Edo discusses how Pinecone can maintain an edge over other incumbents who may build vector database engines into their offerings - check out the queued up video here.
- Katrin, Erik, and Edo share their reasoning about open-source versus closed-source business models -check out the queued up video here.
- Erik opines on open source LLM models being more akin to open science, and whether OpenAI can maintain its lead - check out the queued up video here.
- Edo shares how when Auto GPT came out, Pinecone began having over 10,000 sign ups per day on their site - check out the queued up video here.
- Katrin, Erik, and Edo share their views on Artificial General Intelligence being infinitely far away, 50 years out, or whether it has already arrived. “If you ask academics and machine learning practitioners, and scientists [about] what happened in the last year, we thought it was going to take 30 years.” - check out the queued up video here.
Ready for faster dashboards?
Try for free today.